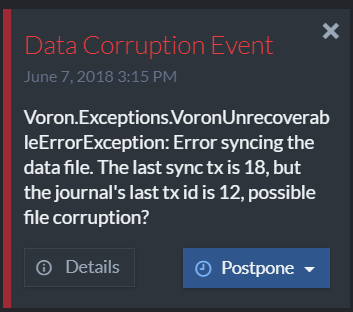
We started to get reports from users that are running RavenDB on Docker that there are situations where RavenDB reports that there has been a data corruption event. You can see how this looks like on the right. As you can see, this ain’t a happy camper. In fact, this is a pretty scary one. The kind you see in movies that air of Friday the 13th.
The really strange part there was that this is one of those errors that really should never be possible. RavenDB have a lot of internal checks, including for things that really aren’t supposed to happen. The idea is that it is better to be safe than sorry when dealing with your data. So we got this scary error, and we looked into it hard. This is the kind of error that gets top priority internally, because it touch at the core of what we do, keeping data safe.
The really crazy part there was that we could find any data loss event. It took a while until we were able to narrow it down to Docker, so we were checking a lot of stuff in the meantime. And when we finally began to suspect Docker, it got even crazier. At some point, we were able to reproduce this more or less at will. Spin a Docker instance, write a lot of data, wait a bit, write more data, see the data corruption message. What was crazy about that was that we were able to confirm that there wasn’t any actual data corruption.
We started diving deeper into this, and it looked like we fell down a very deep crack. Eventually we figured out that you need the following scenario to reproduce this issue:
- A Linux Docker instance.
- Hosted on a Windows machine.
- Using an external volume to store the data.
That led us to explore exactly how Docker does volume sharing. I a Linux / Linux or Windows / Windows setup, that is pretty easy, it basically re-route namespaces between the host and the container. In a Linux container running on a Windows machine, the external volume is using CIFS. In other words, it is effectively running on a network drive, even if the network is machine local only.
It turned out that the reproduction is not only very specific for a particular deployment, but also for a particular I/O pattern.
The full C code reproducing this can be found here. It is a bit verbose because I handled all errors. The redacted version that is much more readable is here:
This can be executed using:
And running the following command:
docker run --rm -v PWD:/wrk gcc /wrk/setup.sh
As you can see, what we do is the following:
- Create a file and ensure that it is pre-allocated
- Write to the file using O_DIRECT | O_DSYNC
- We then read (using another file descriptor) the data
The write operations are sequential, and the read operations as well, however, the read operation will read past the written area. This is key. At this point, we write again to the file, to an area where we already previously read.
At this point, we attempt to re-read the data that was just written, but instead of getting the data, we get just zeroes. What I believe is going on is that we are hitting the cached data. Note that this is doing system calls, not any userland cache.
I reported this to Docker as a bug. I actually believe that this will be the same whenever we use CIFS system (a shared drive) to run this scenario.
The underlying issue is that we have a process that reads through the journal file and apply it, at the same time that transactions are writing to it. We effectively read the file until we are done, forcing the file data into the cache. The writes, which are using direct I/O are going to bypass that cache and we are going to have to wait for the change notification from CIFS to know that this needs to be invalidated. That turn this issue into a race condition of data corruption,of sort.
The reason that we weren’t able to detect data corruption after the fact was that there was no data corruption. The data was properly written to disk, we were just mislead by the operating system about that when we tried to read it and got stale results. The good news is that even after catching the operating system cheating on us with the I/O system, RavenDB is handling things with decorum. In other words, we immediately commit suicide on the relevant database. The server process shuts down the database, register an alert and try again. At this point, we rely on the fact that we are crash resistant and effectively replay everything from scratch. The good thing about this is that we are doing much better the second time around (likely because there is enough time to get the change event and clear the cache). And even if we aren’t, we are still able to recover the next time around.
Running Linux containers on Windows is a pretty important segment for us, developers using Docker to host RavenDB, and it make a lot of sense they will be using external volumes. We haven’t gotten to testing it out, but I suspect that CIFS writes over “normal” network might exhibit the same behavior. That isn’t actually a good configuration for a database for a lot of other reasons, but that is still something that I want to at least be able to limp on. Even with no real data loss, a error like the one above is pretty scary and can cause a lot of hesitation and fear for users.
Therefor, we have changed the way we are handling I/O in this case, we’ll avoid using the two file descriptors and hold a bit more data in memory for the duration. This give us more control, actually likely to give us a small perf boost and avoid the problematic I/O pattern entirely.